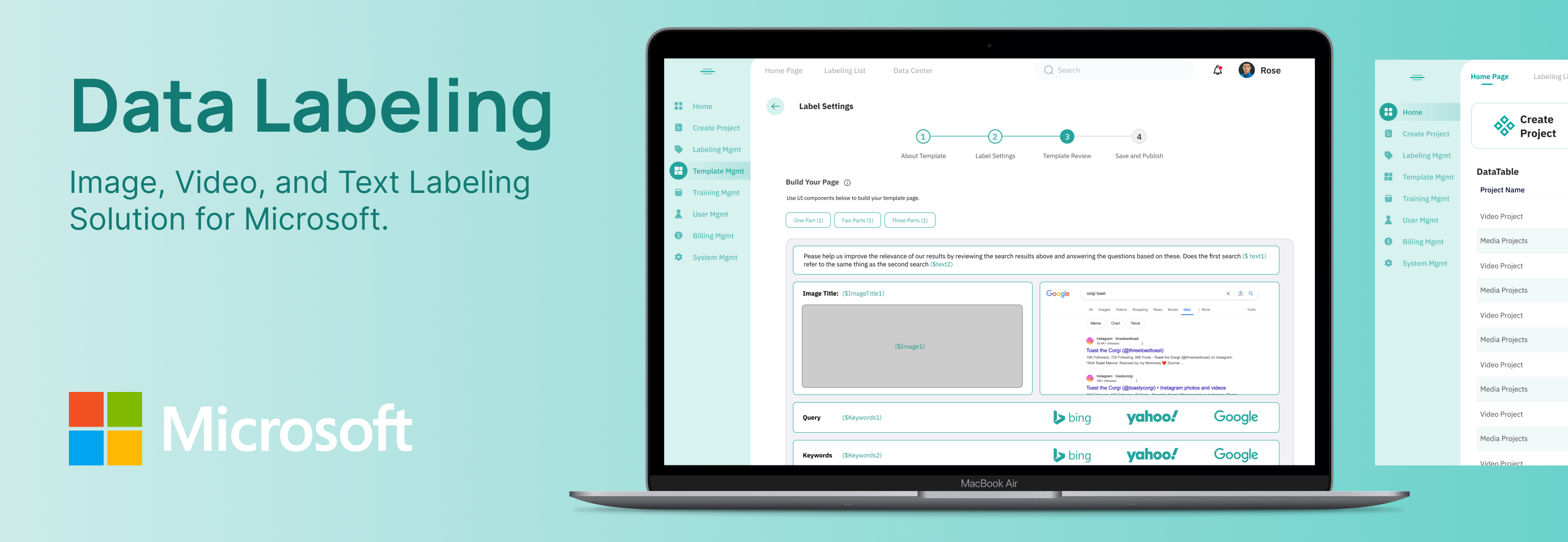
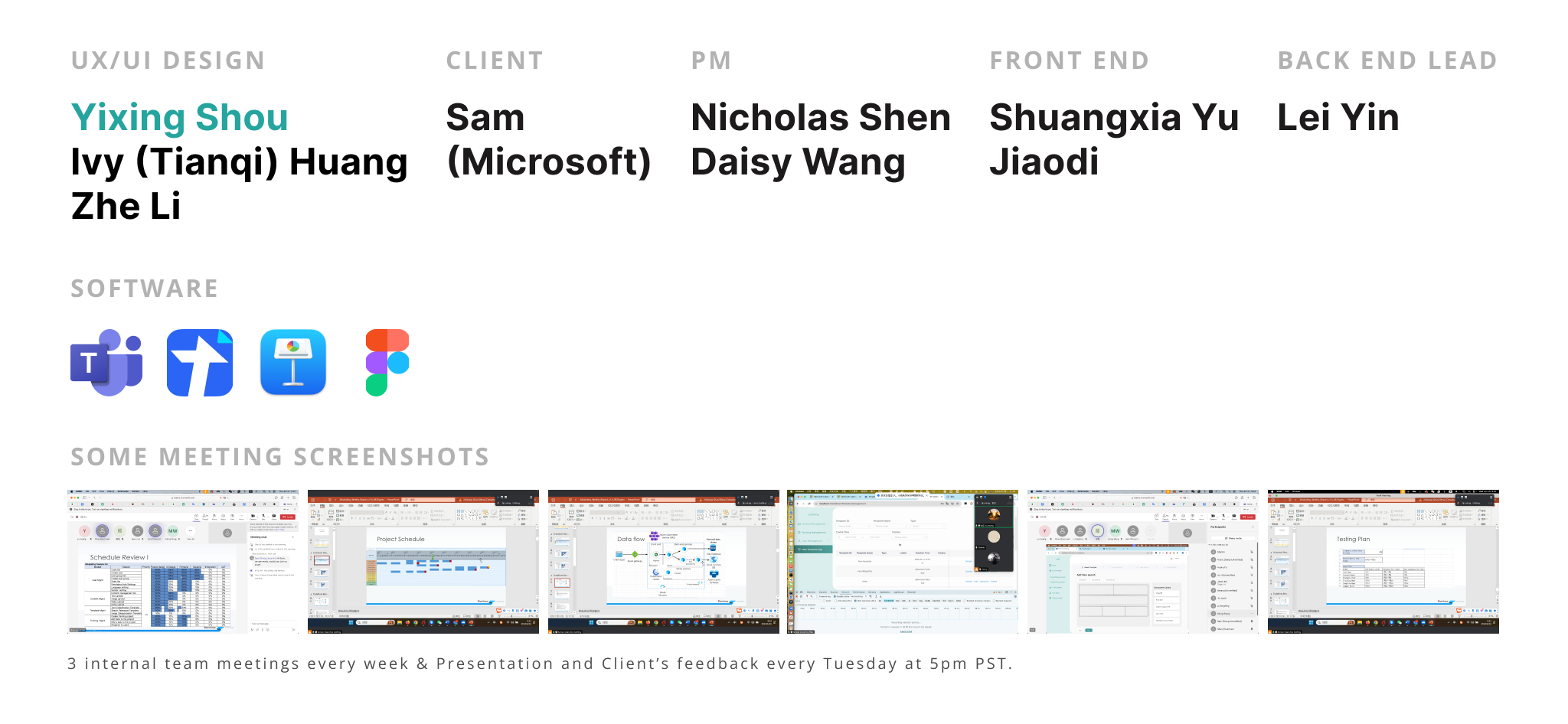
Timeline
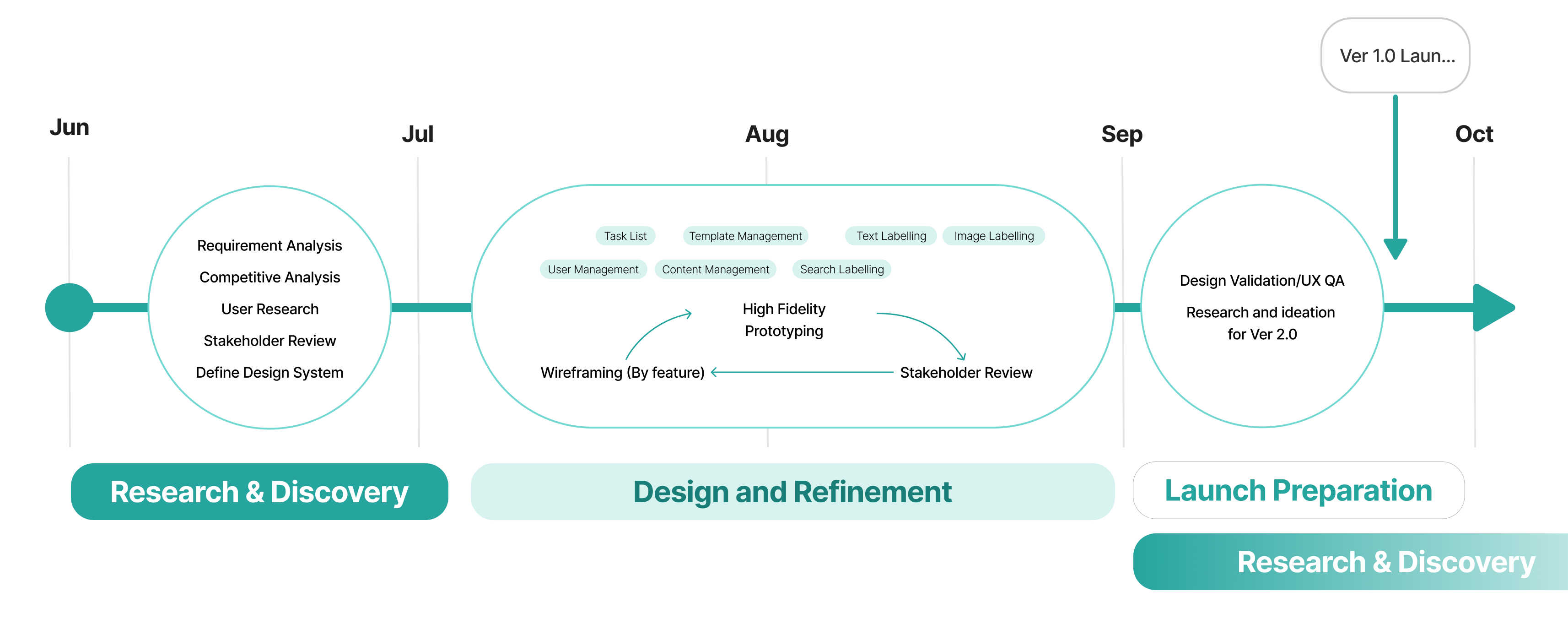
As a team with 20+ members in total, we communicate needs, design, Technical Implementation by meetings. In average, we have internal meetings 3 times a week and presentation to our client every Wednesday. We have a shared sheet for all teams to update real-time progress.
Solution Overview
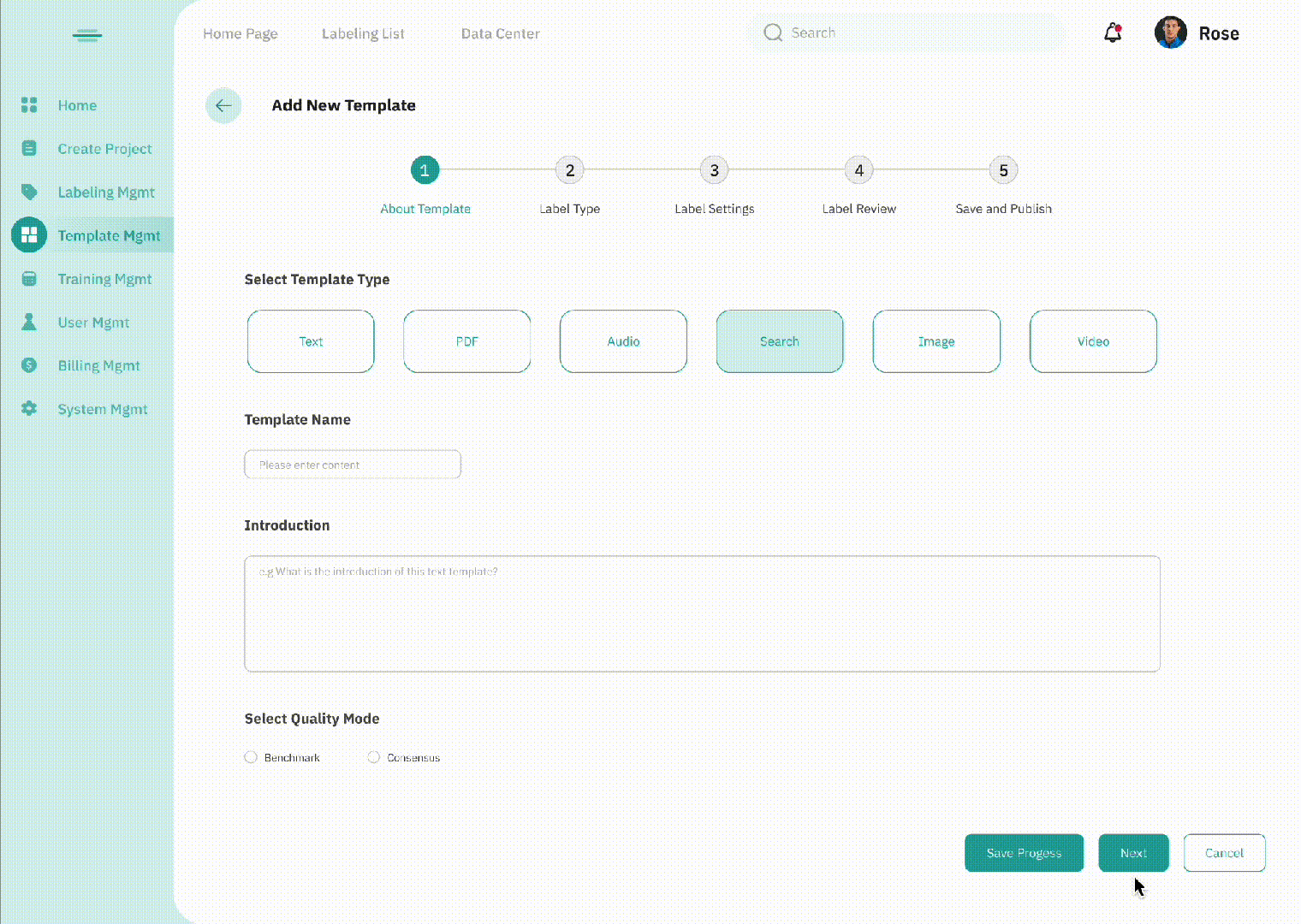
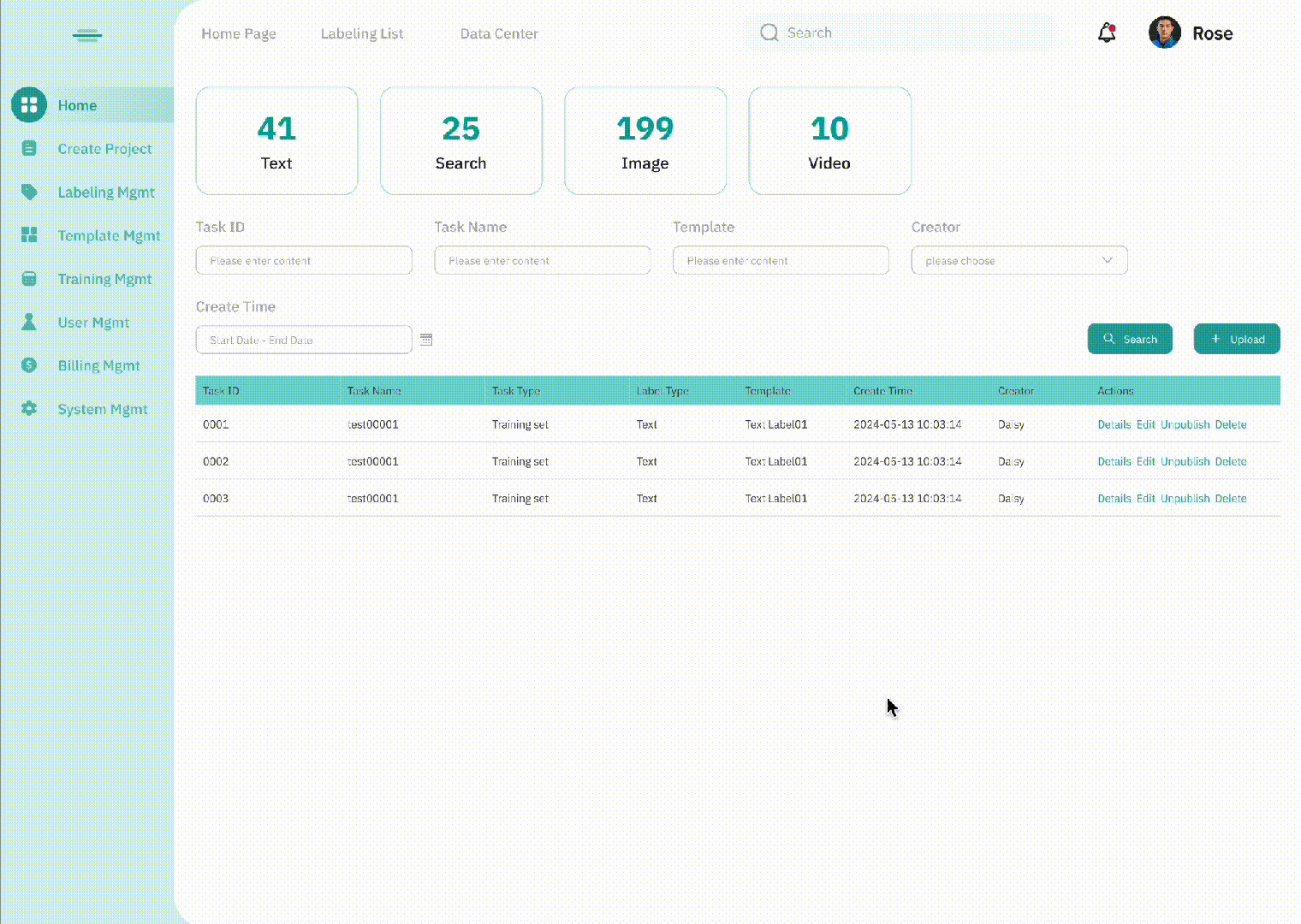
This product for Microsoft streamlines the annotation of image, text, and search data for machine learning.
By automating tasks like tagging images, categorizing text, and refining search results, it reduces manual effort, speeds up AI model training, and lowers operational costs.
Understand AI Labeling and Deep Learning
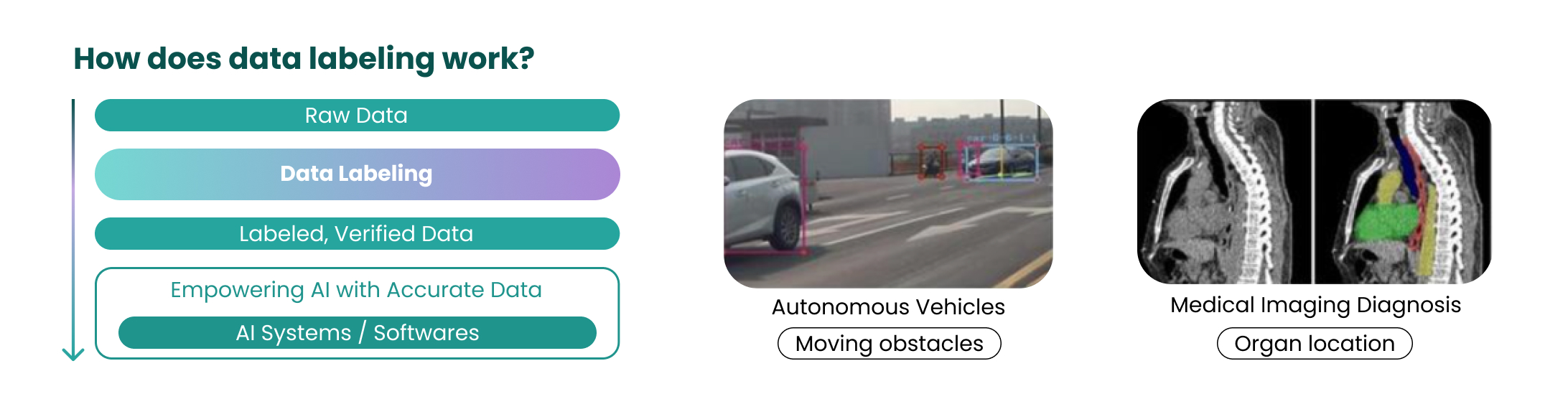
To better understand AI's role in medical diagnosis, I interviewed thoracic surgeons, Dr. Pei and Dr. Huang. Their insights into cancer diagnosis workflows and CT labeling processes informed the design of a more user-friendly labeling feature.
“AI in diagnostics helps conserve medical resources.(The time doctors spend on diagnosis)” --Dr. Huang
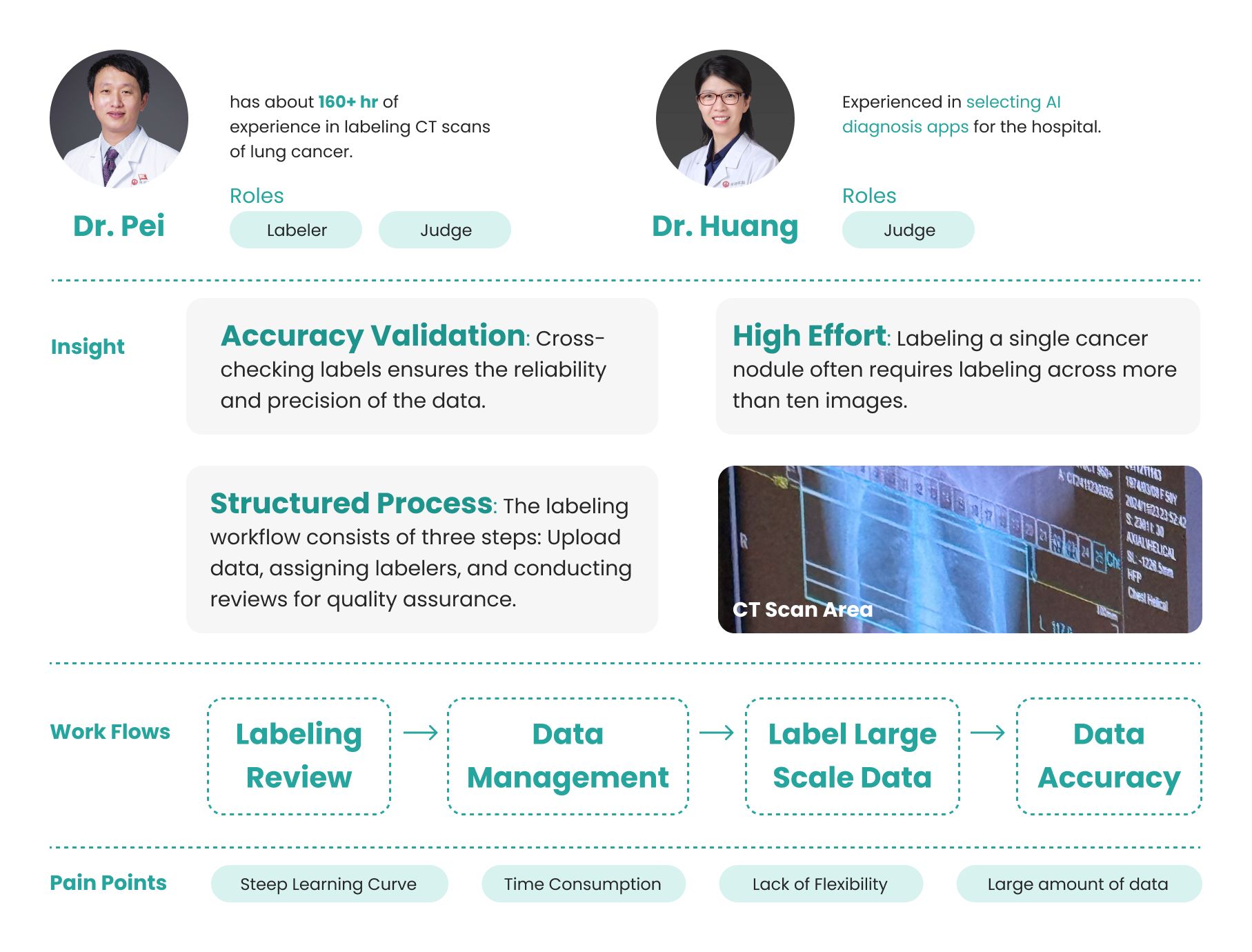
(Interview: Click here to Read more)
Research: Competitive Analysis
To begin our competitive analysis, our team collaborated with stakeholders to identify the need for supporting multiple data types, including image, text, and search labeling, for our MVP. Partnering with the product manager, I researched platforms like Labelbox, AWS, and Scale AI to evaluate their strengths in data support and user experience. Insights from this analysis helped us prioritize key features and shape the user experience design for our MVP.
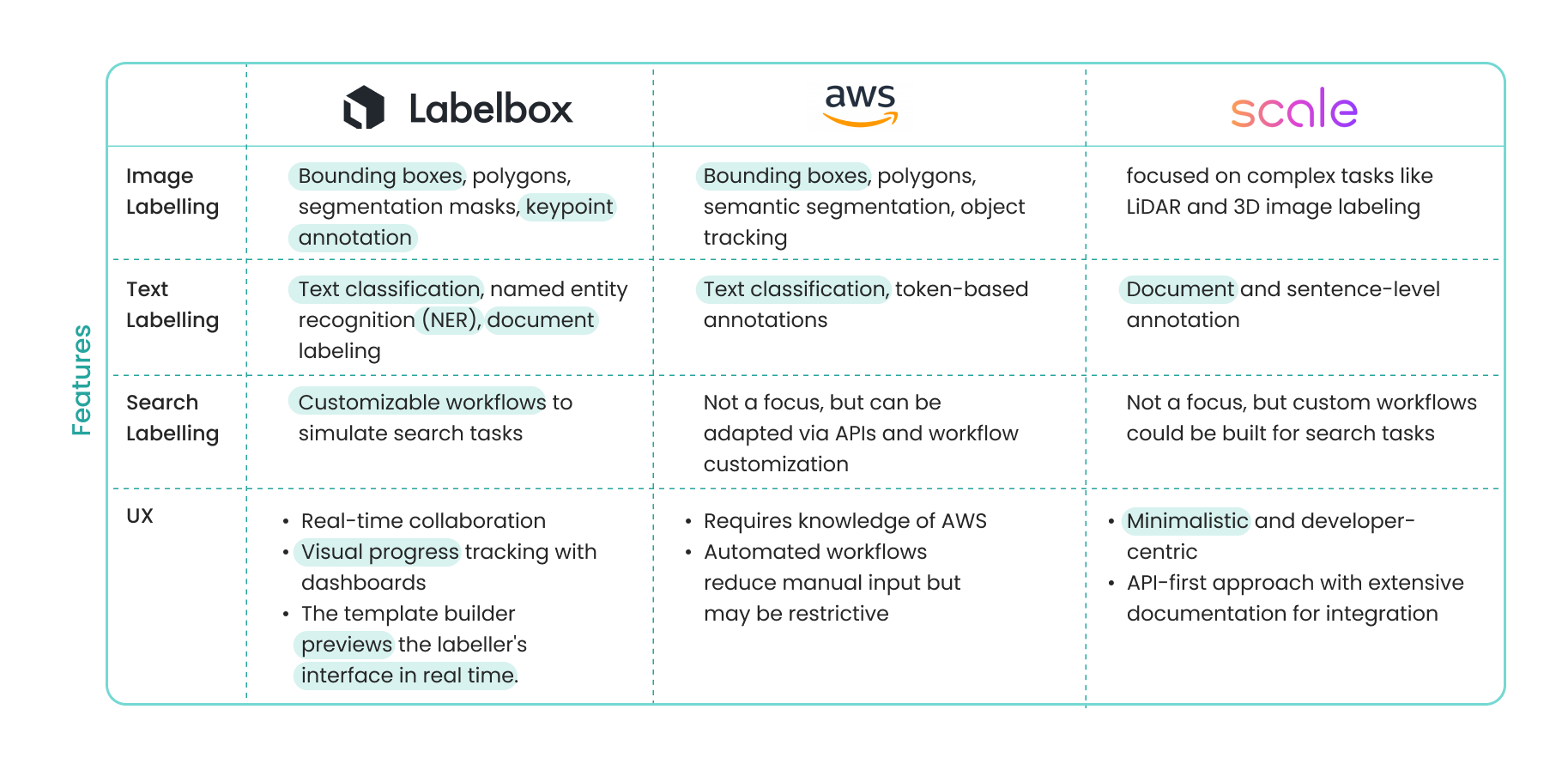
Ideation: Collecting Ideas
During team meetings with product managers, affinity mapping has been incredibly useful for organizing ideas gathered from our research, as well as client feedback. For example, we use sticky notes to capture insights from different sources, then rearrange them into sections. This method helps us identify key features and ultimately develop a feature list.
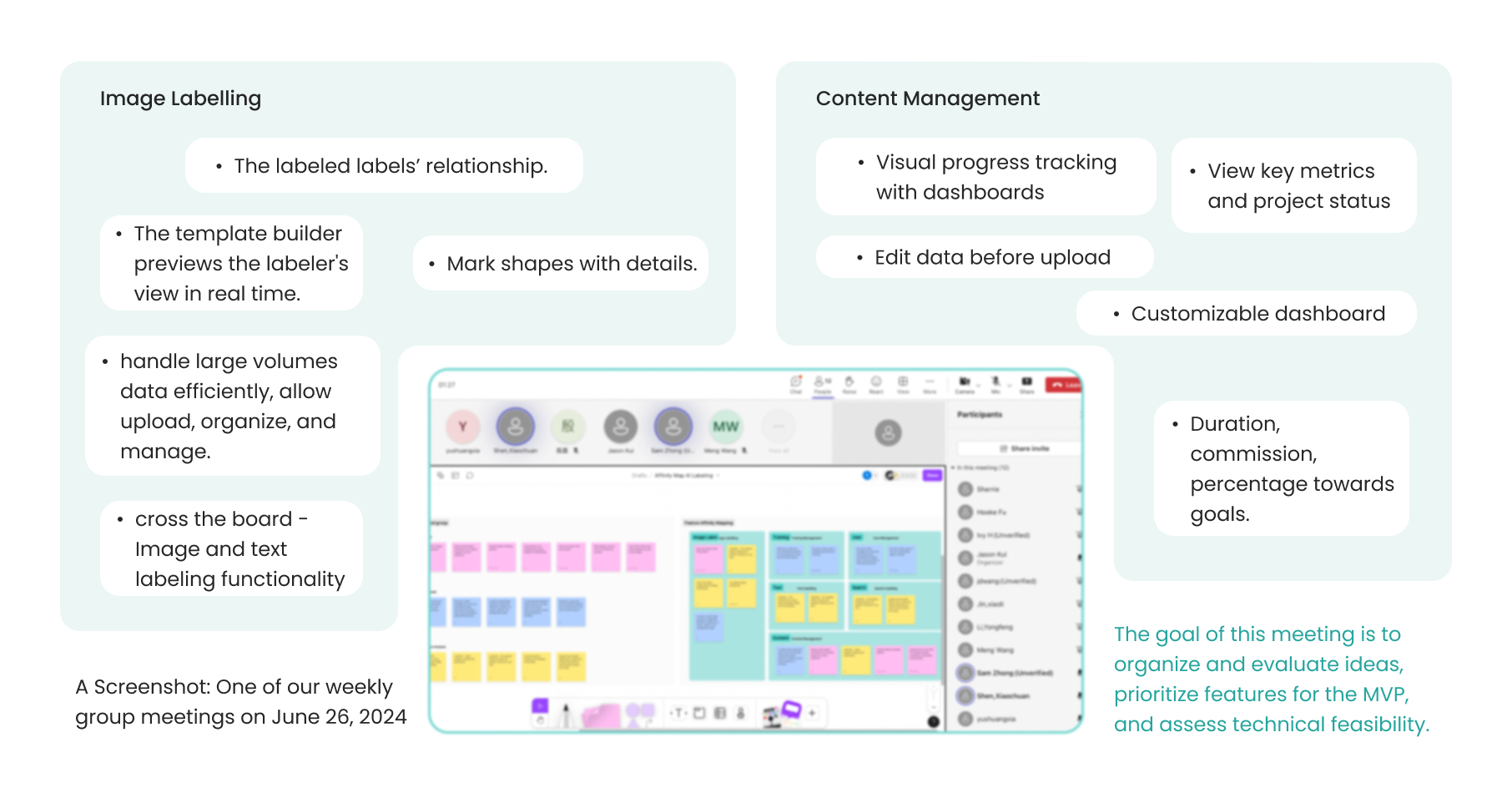
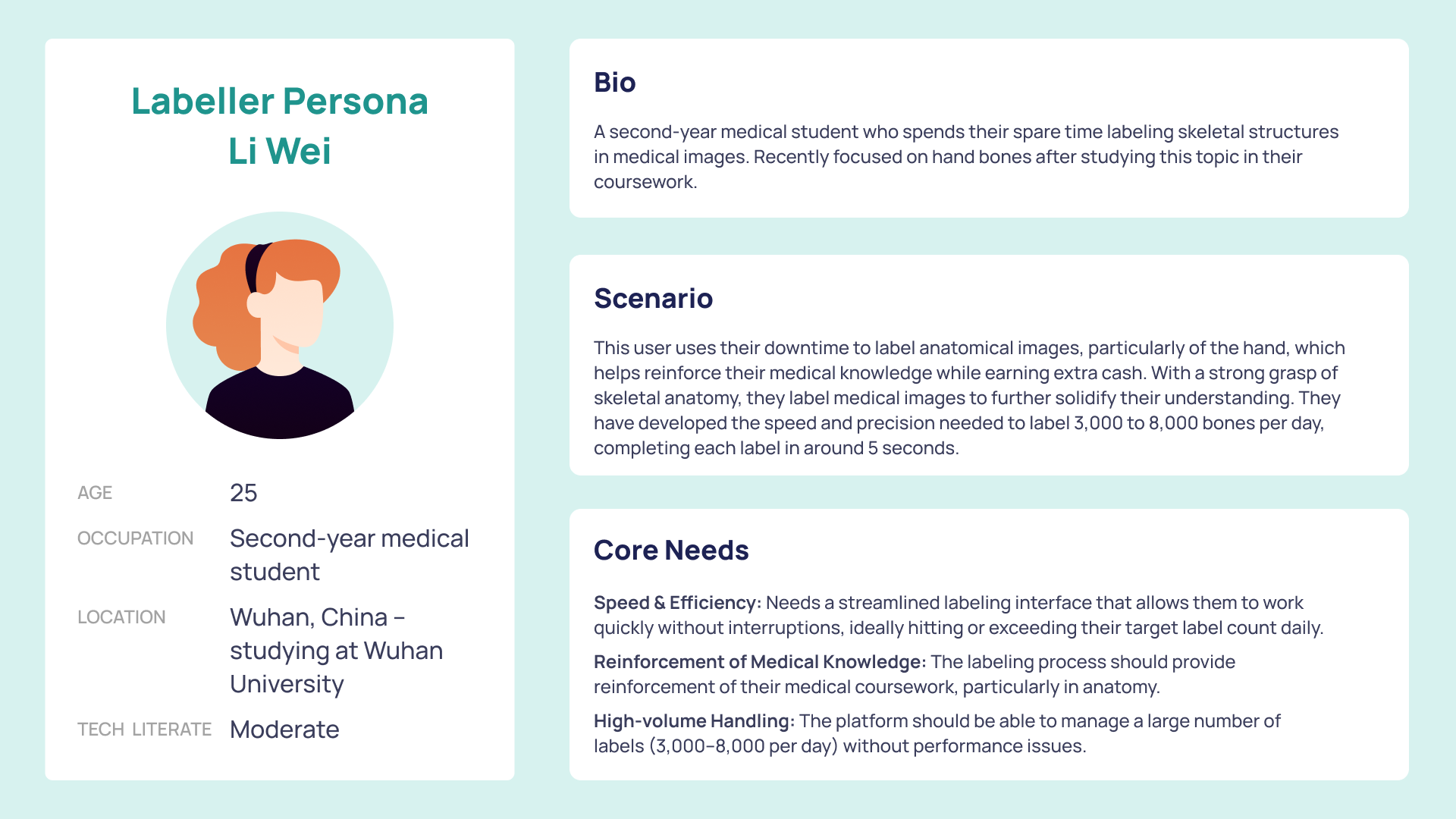
Daisy (our Product Manager), Ivy, and I collaborated on developing feature map, user flows and wireframes. For each feature, we carefully considered the user journey, streamlining the process to include only the essential steps. Below are examples of the user flows and wireframes we created.
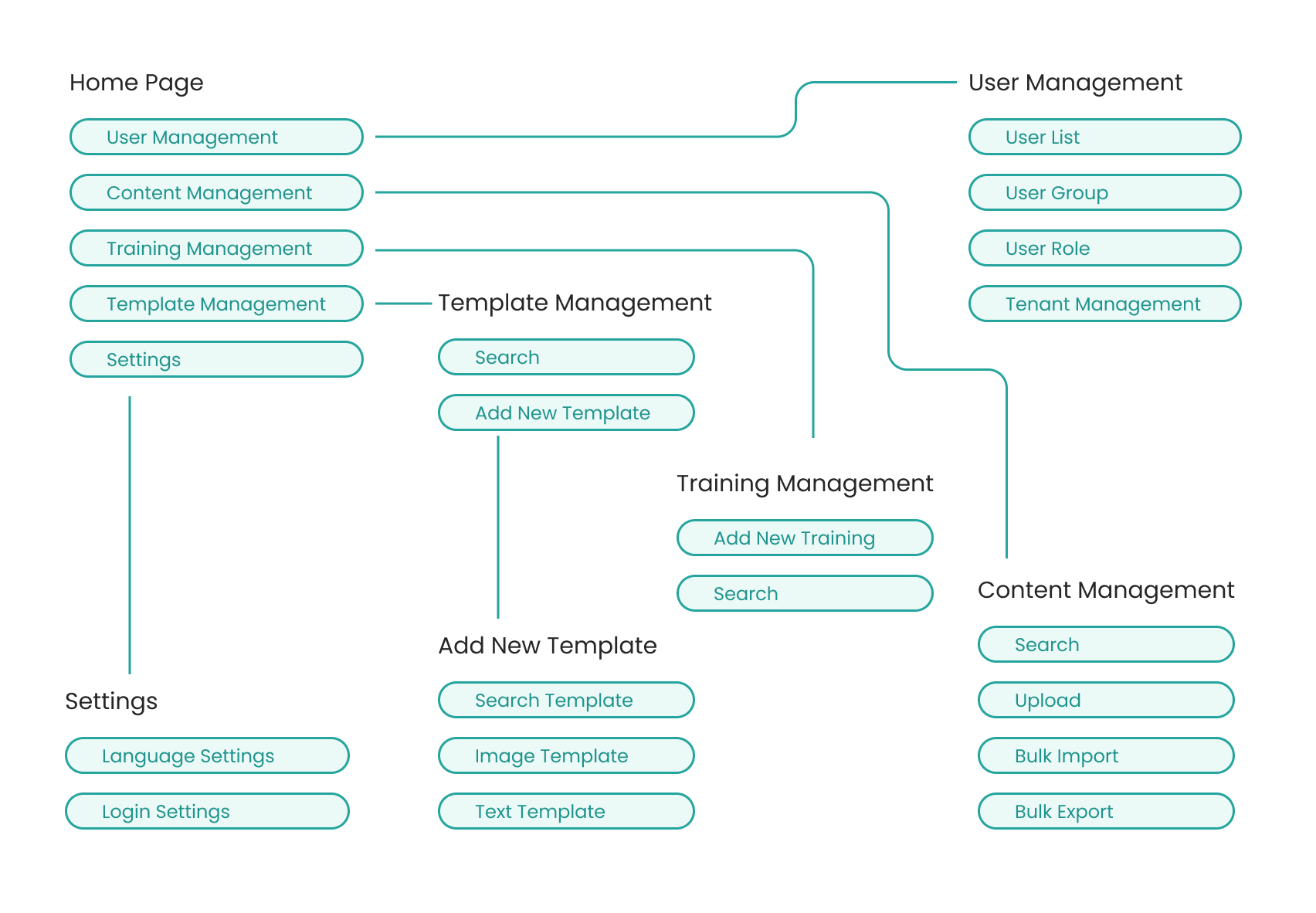
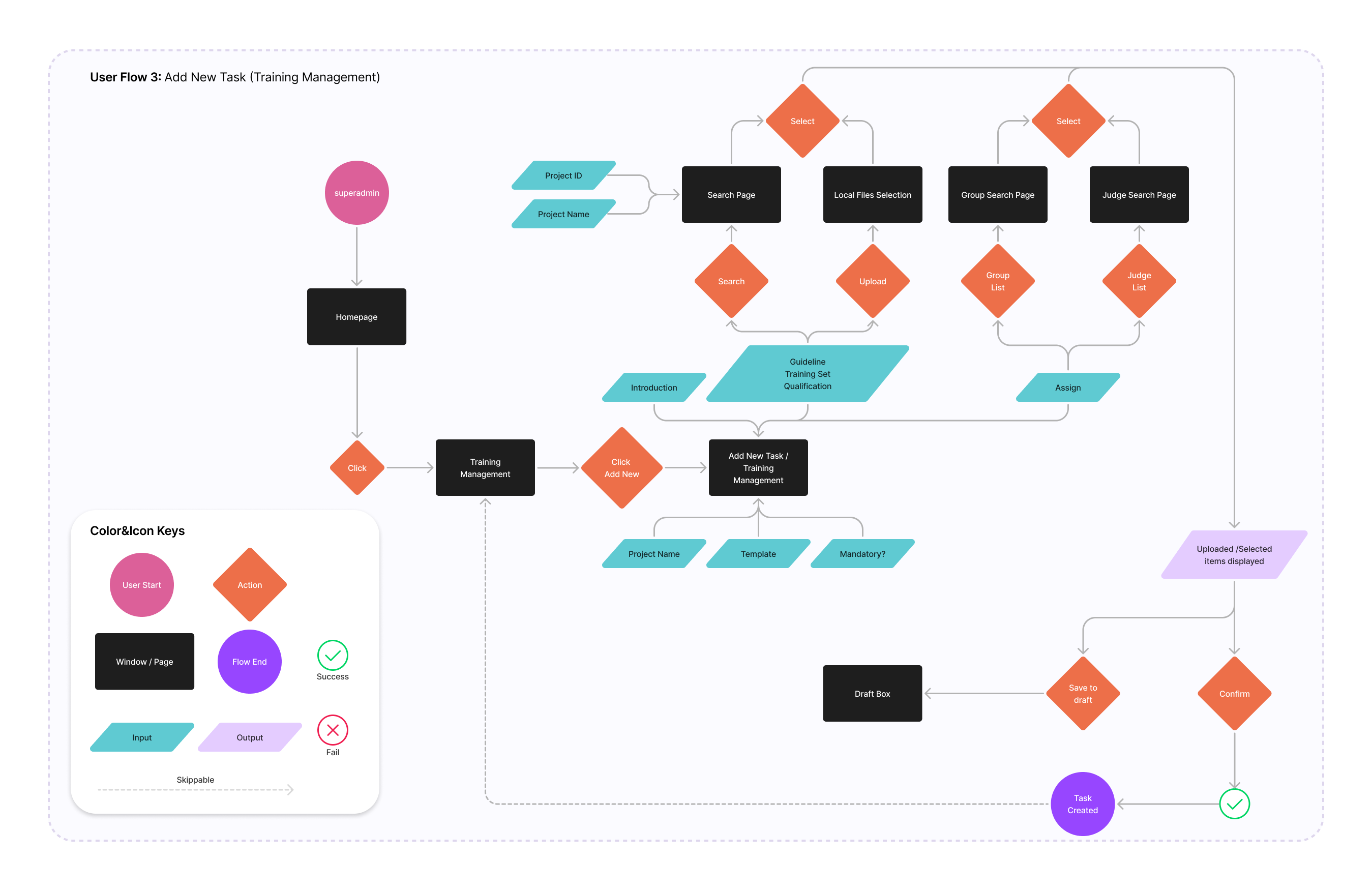
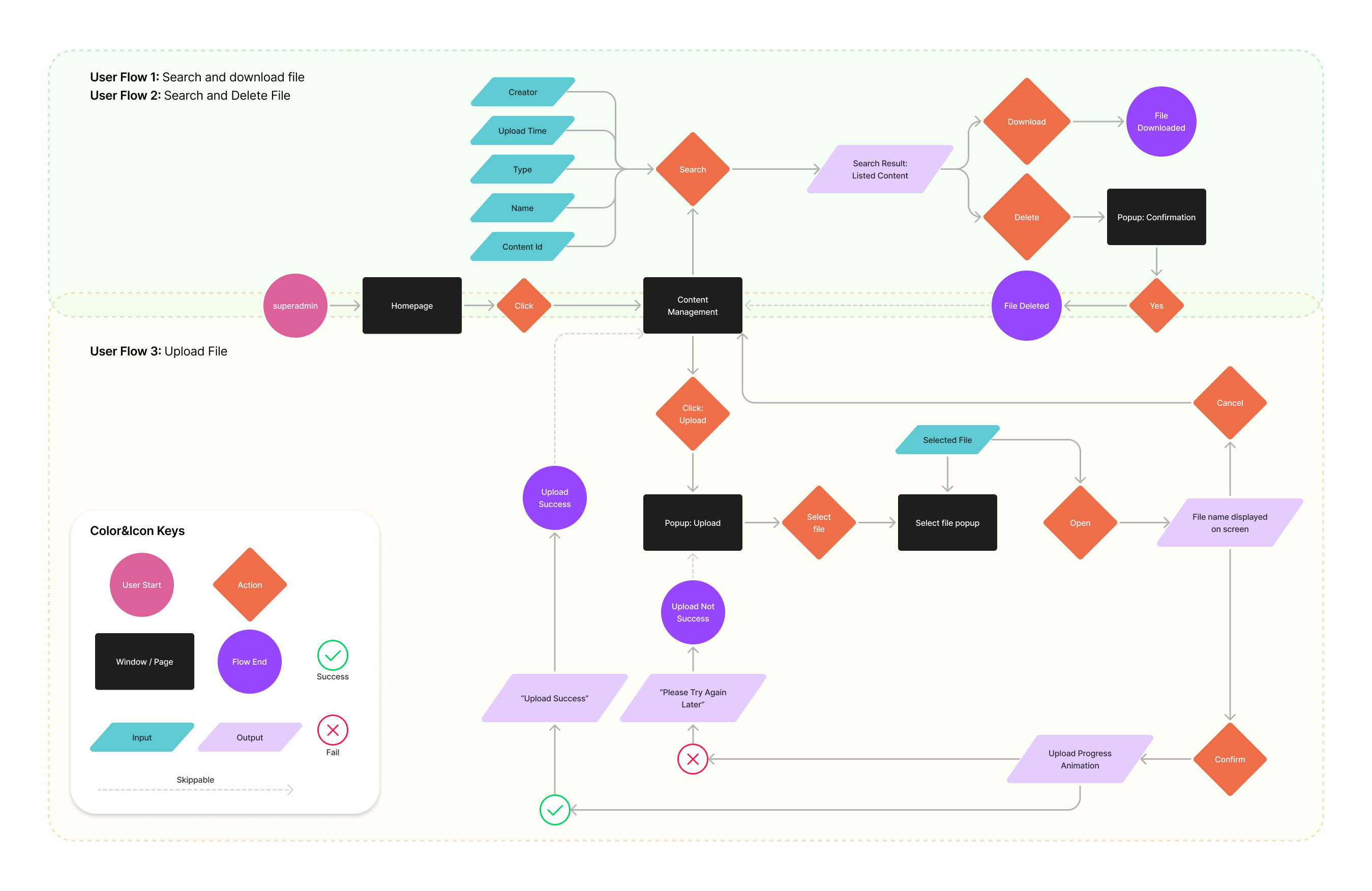
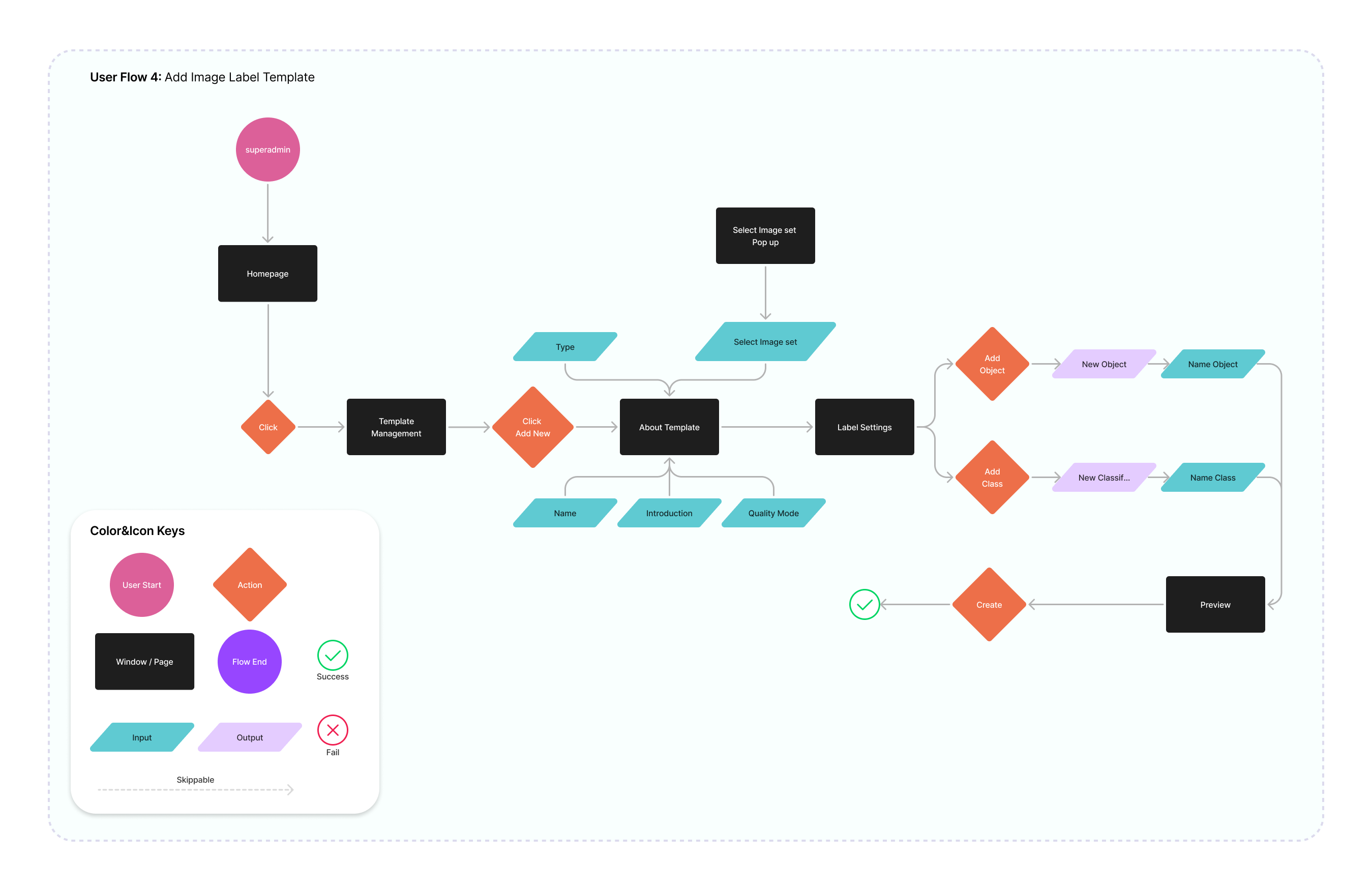
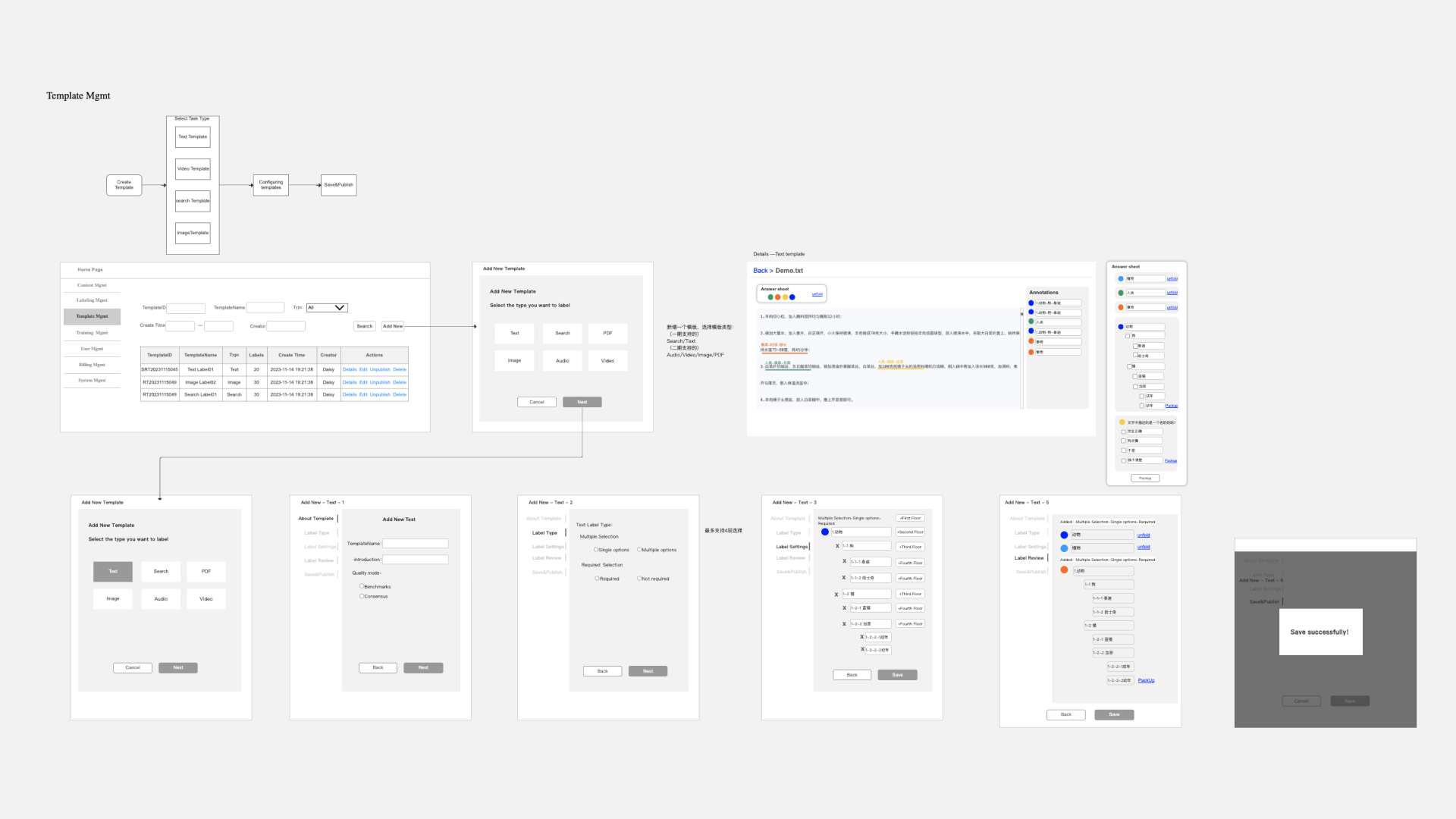
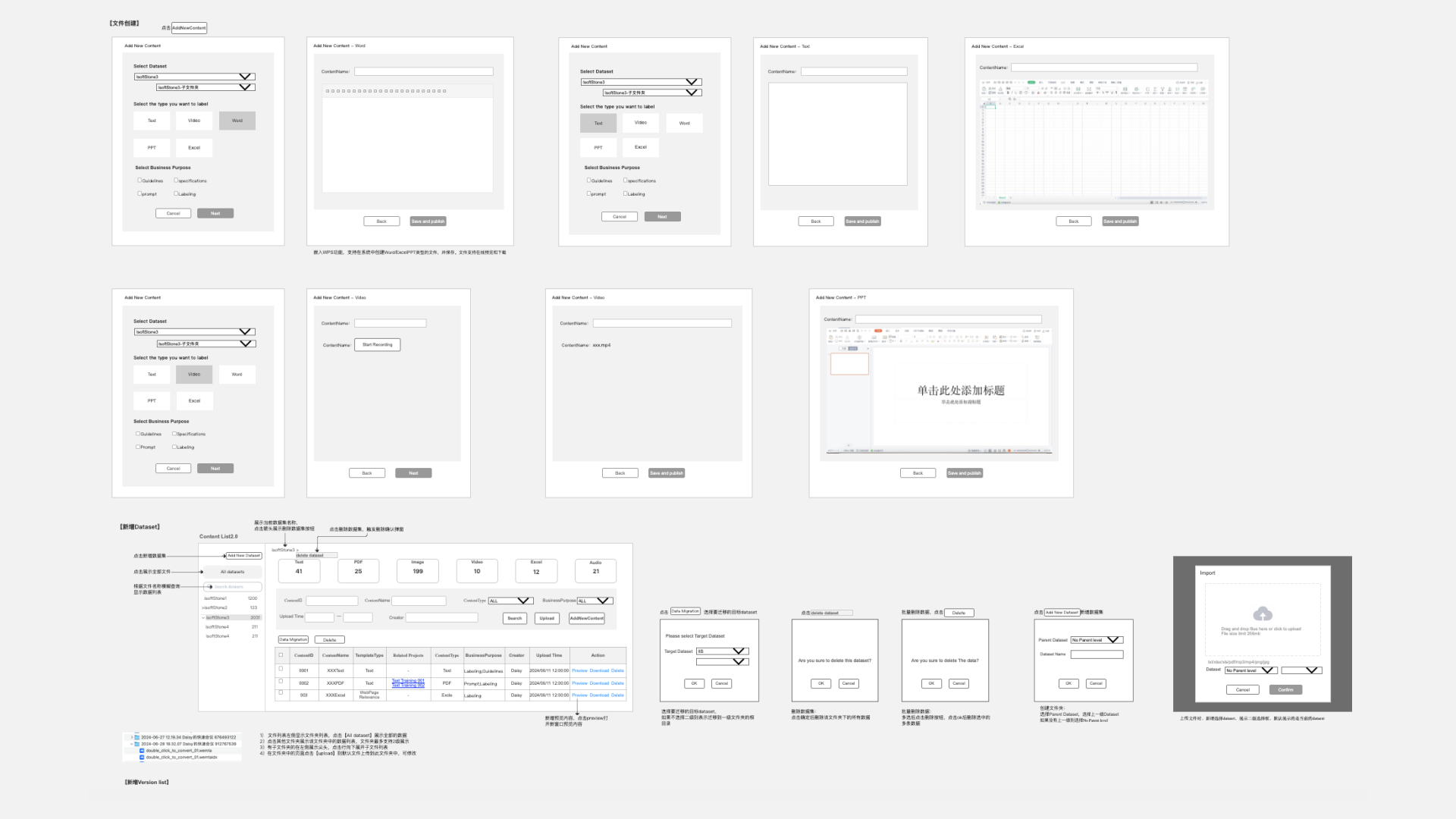
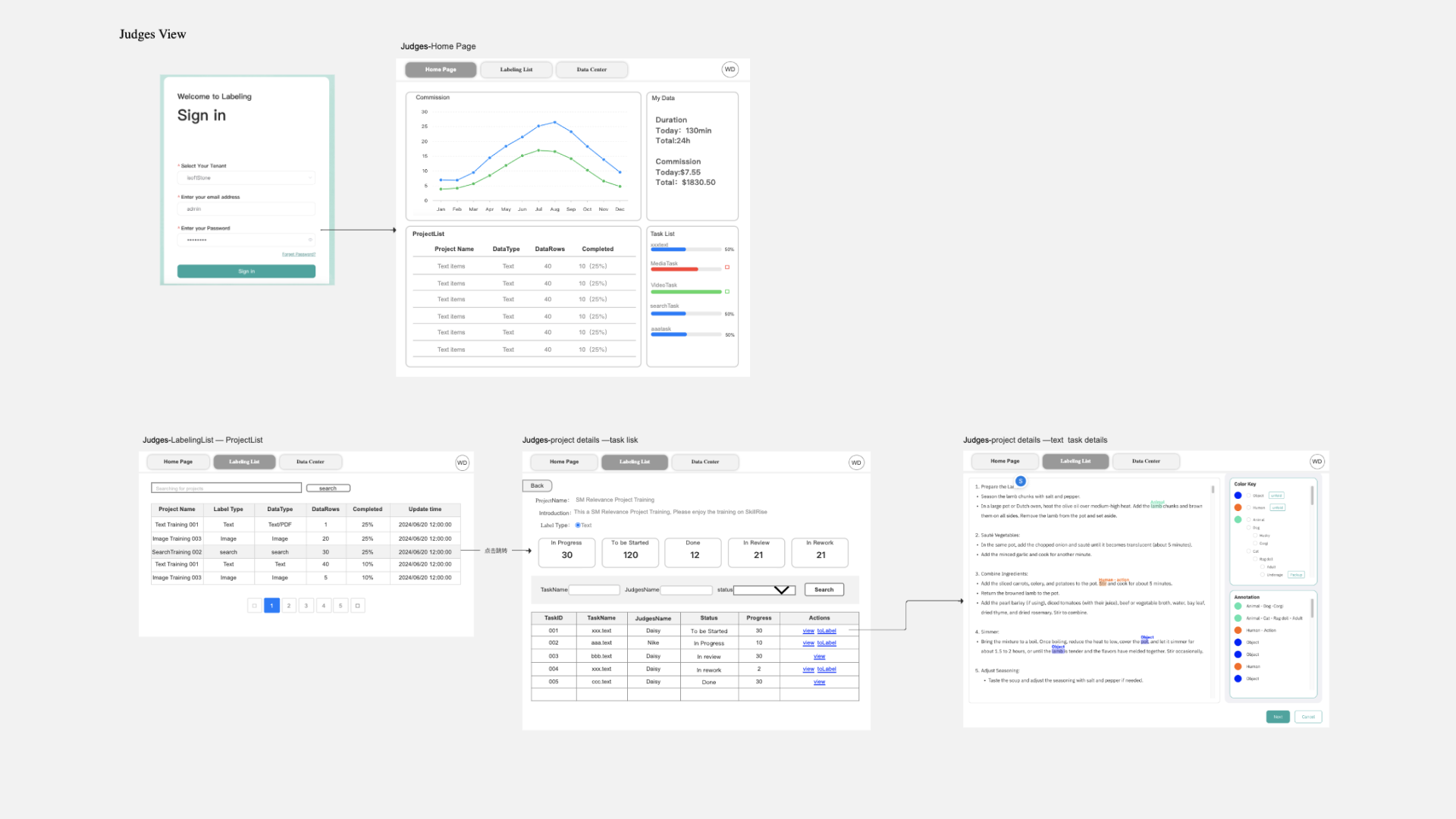
UI Kit
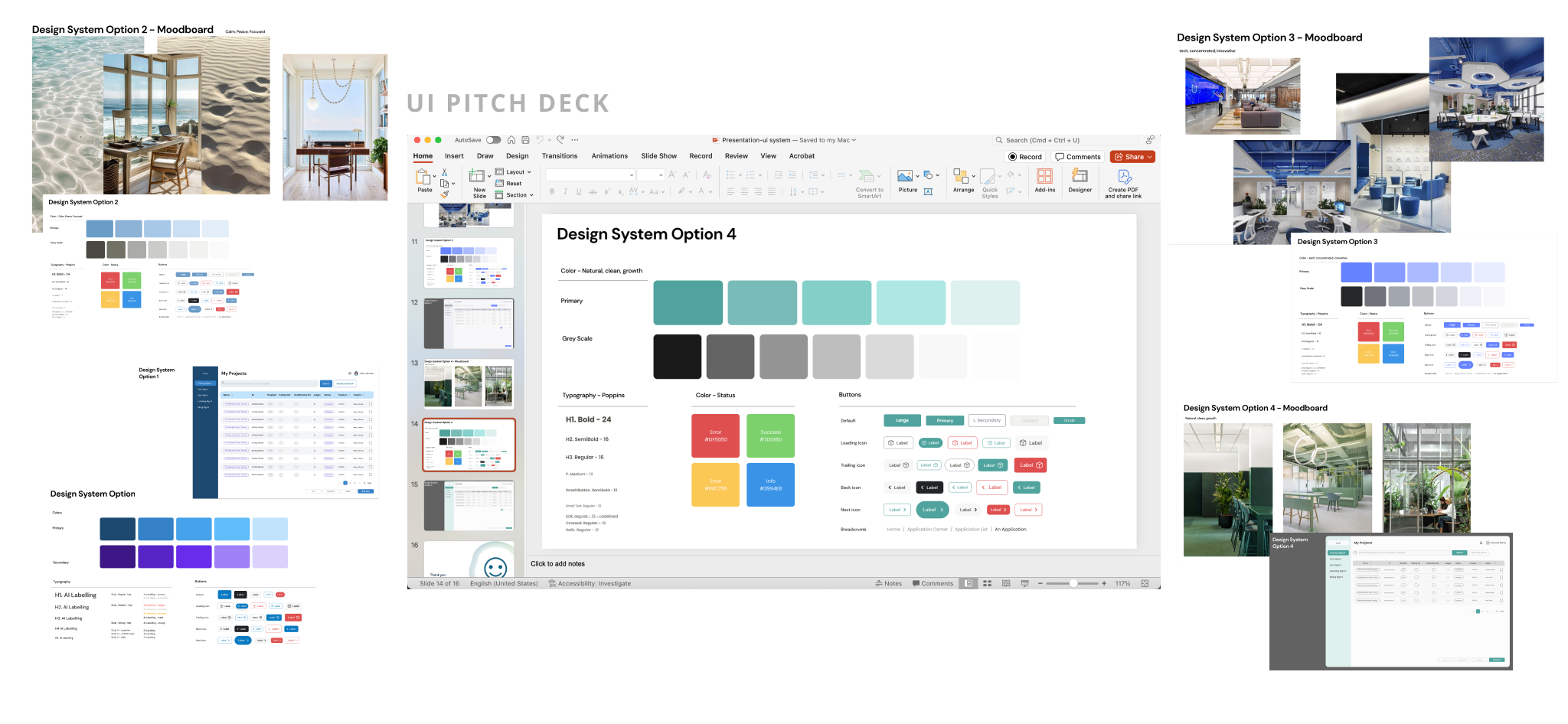
We have provided Sam (our client) 4 design options with moodboard and mockups, and Sam picked the monochrome green / teal color scheme.
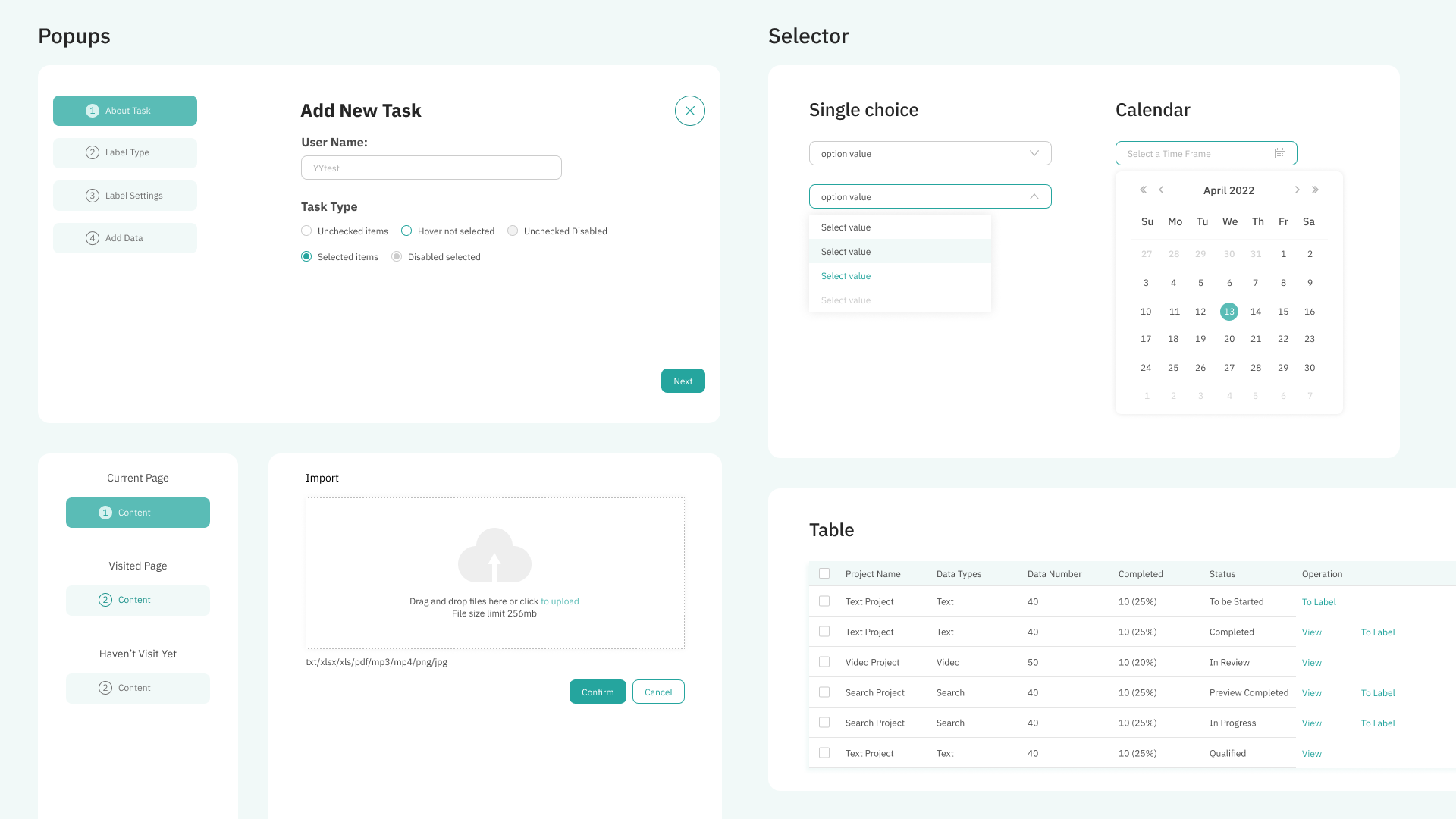
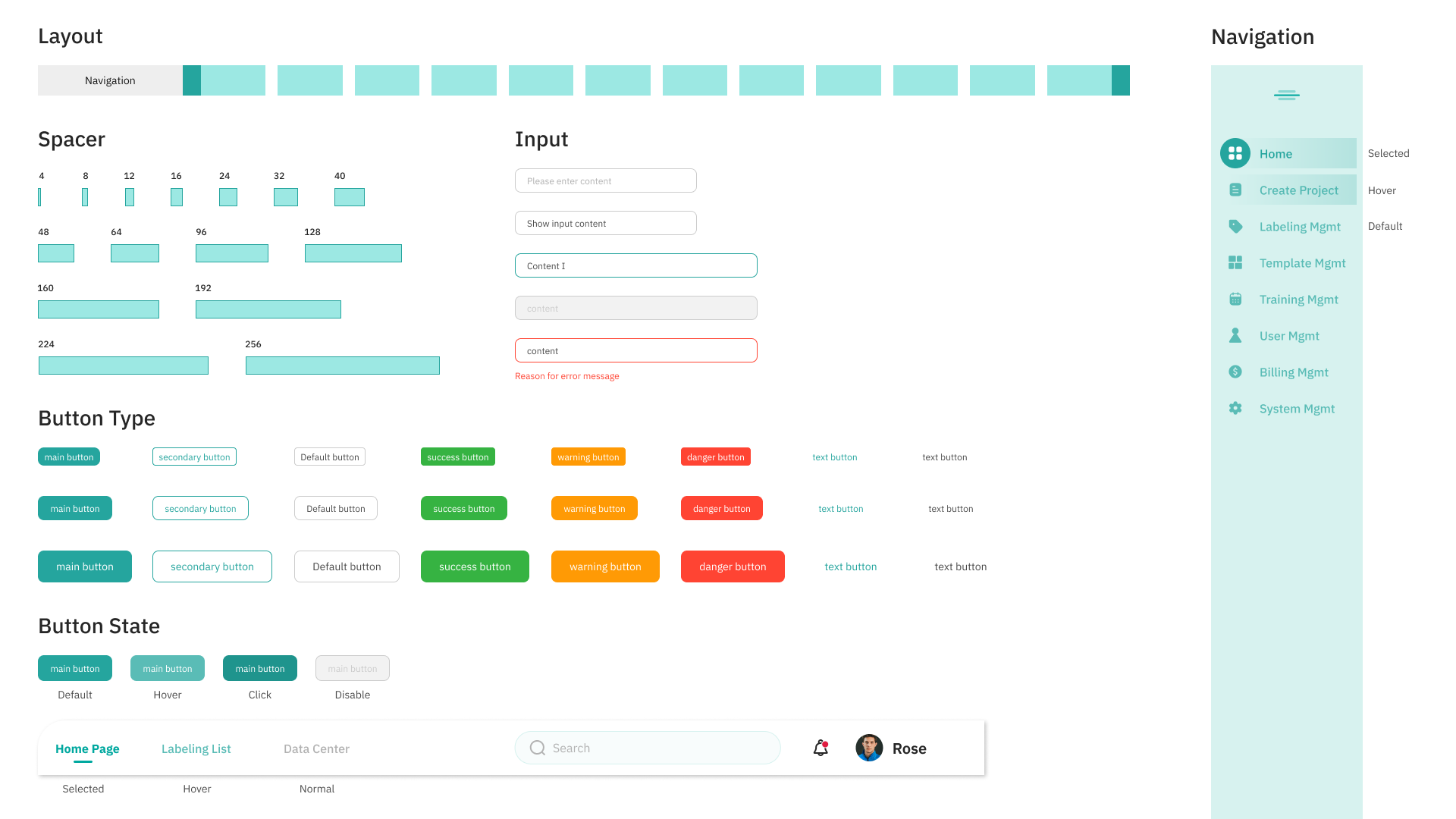
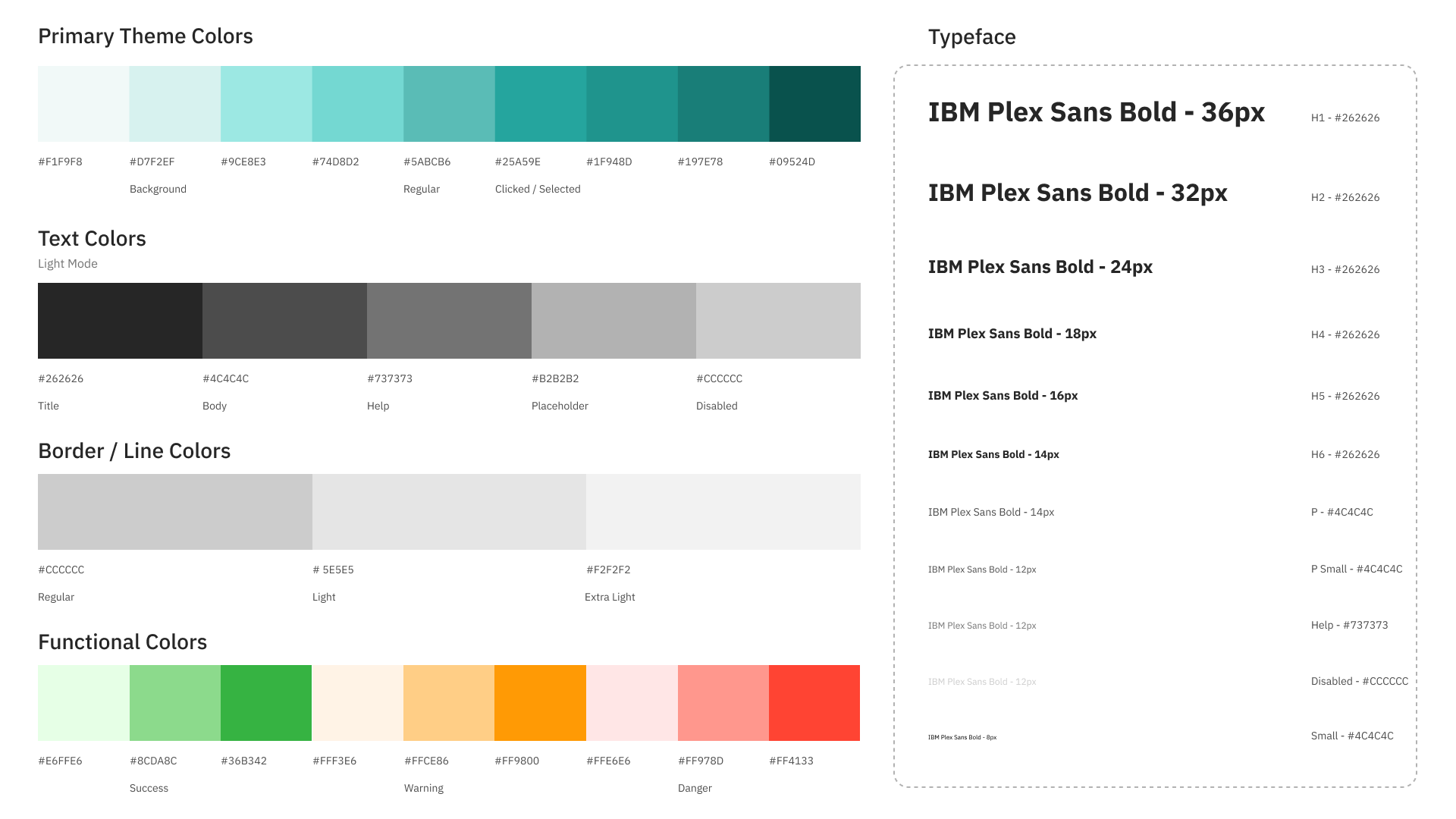
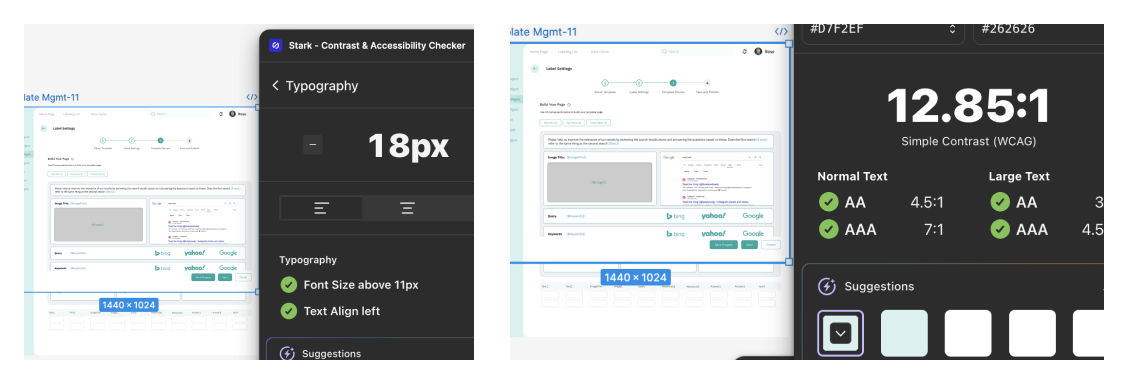
Feature Breakdown (Image labeling)
While we recognize that judges are already familiar with creating templates, our goal was to provide a modular and intuitive interface that further simplifies their workflow. We have annotated key features developed based on both user and client needs.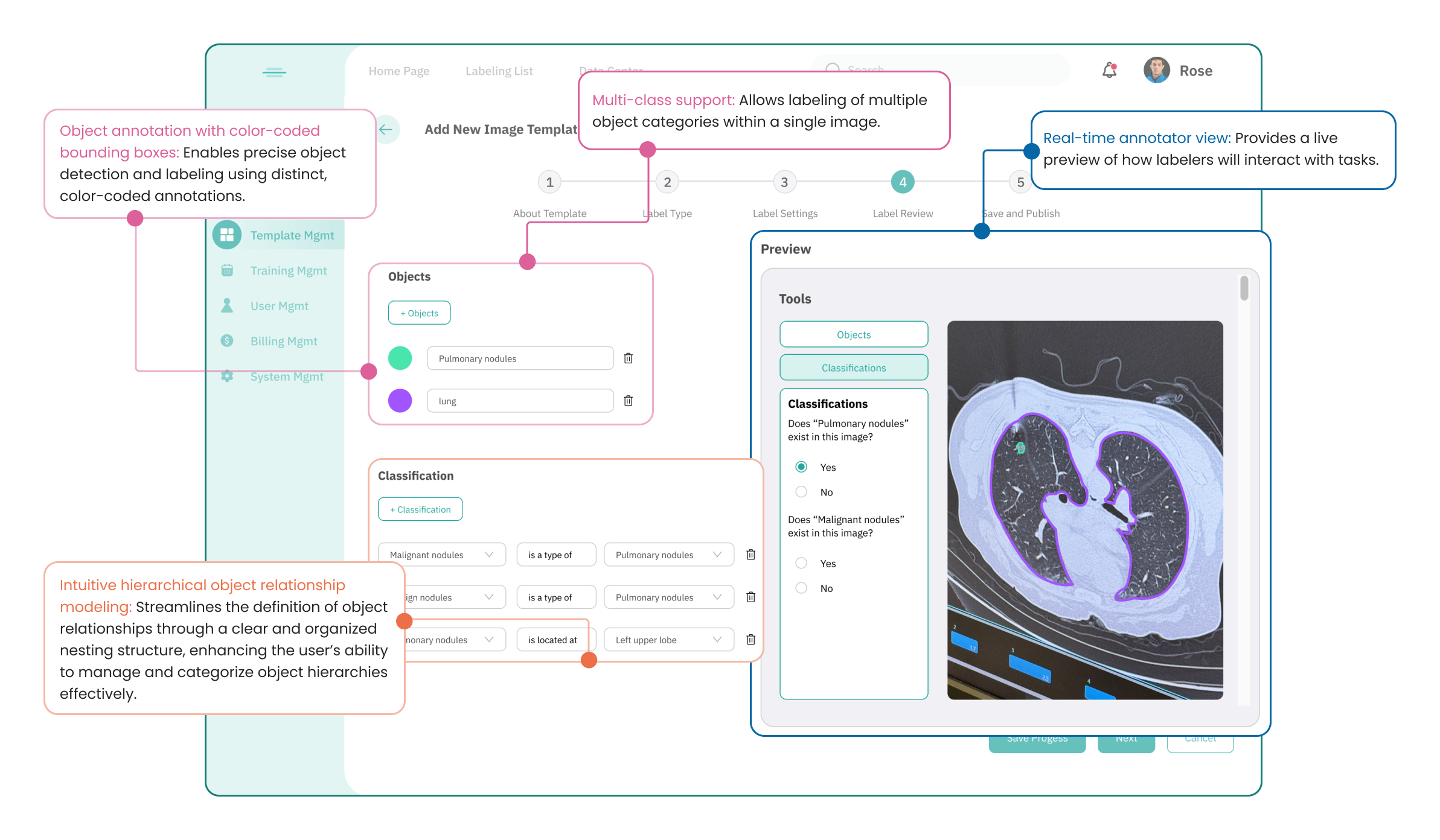
Design Validation: UI Walk Through
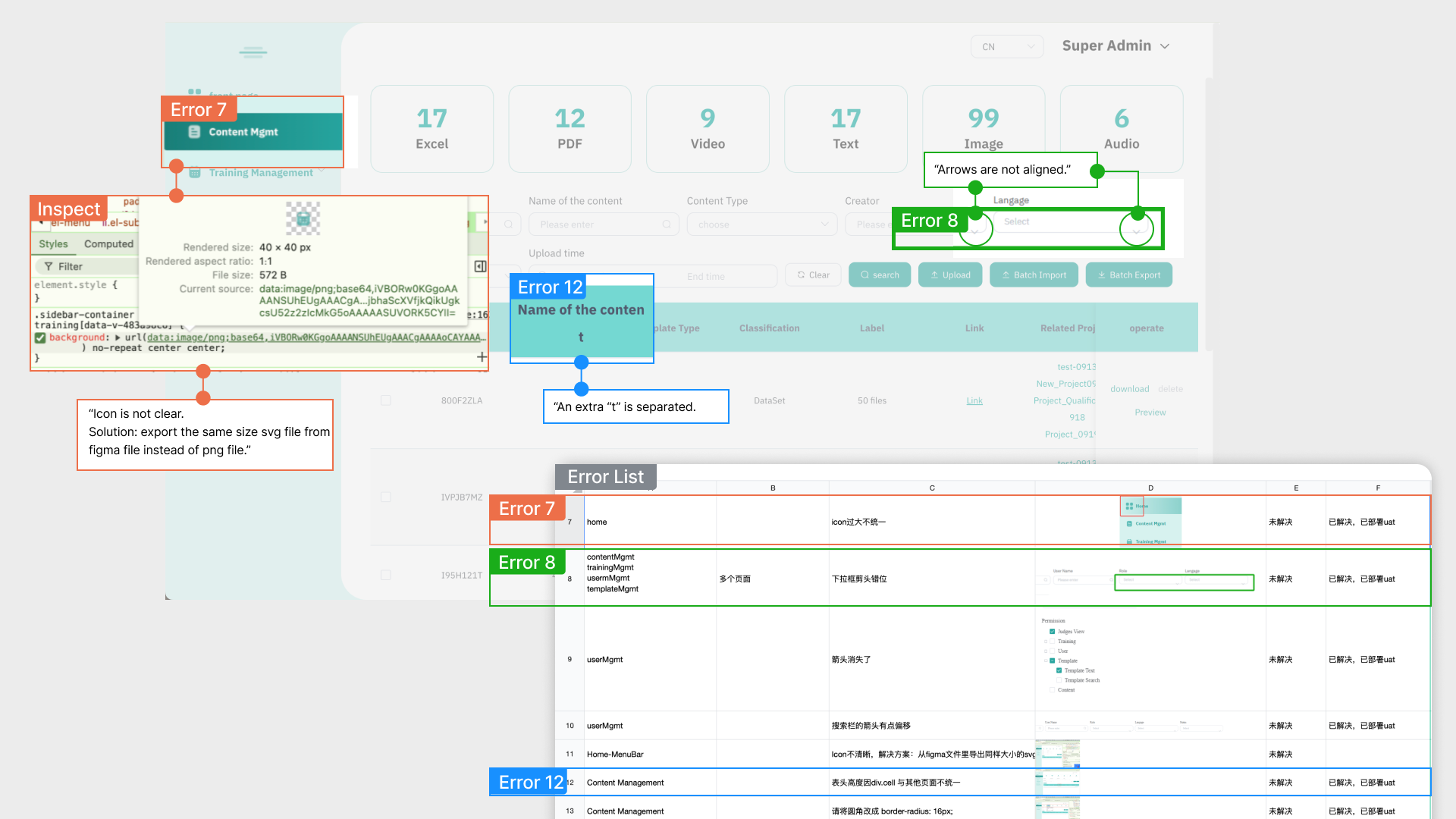
Given the tight development timeline, the front-end engineers began development as we were still working on the high-fidelity prototype. This allowed us, as designers, to conduct timely walkthroughs and identify areas for improvement. To streamline the review process and ensure clear communication, we set up a shared Excel sheet between the design and front-end teams to track page modifications. This enabled us to provide timely feedback and synchronize updates after each revision.
Usability Testing
Feedback is essential for refining our product, addressing pain points, and enhancing the overall user experience. Below are sample questions designed to gather insights into the platform’s technical functionality and its effectiveness in supporting labeling tasks.
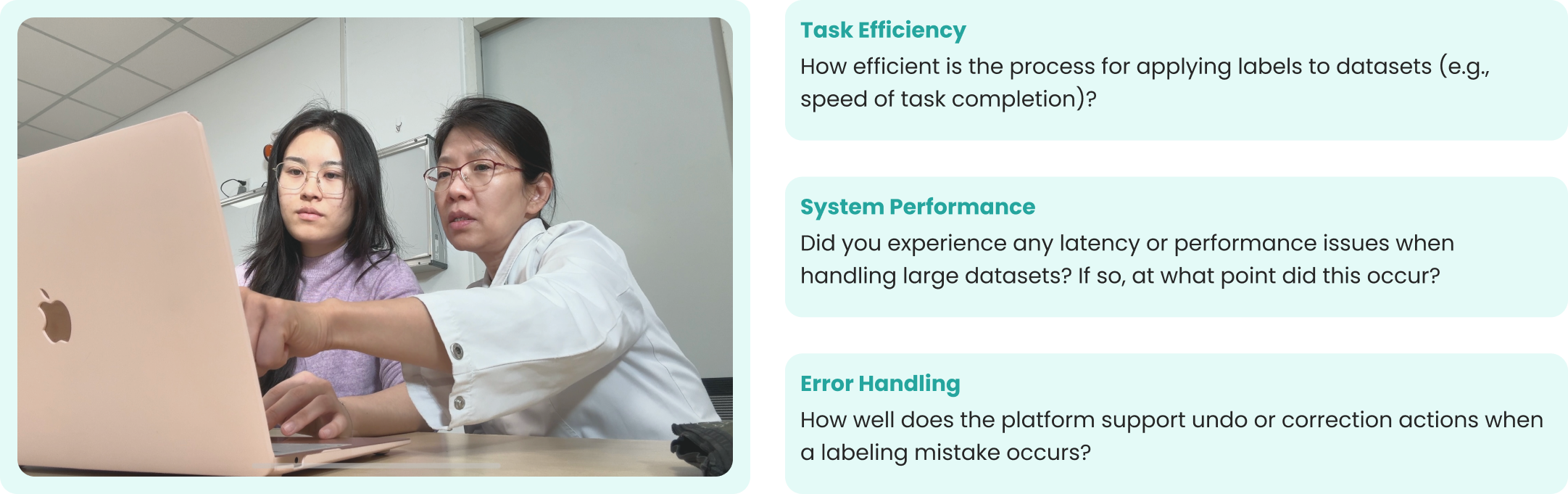
Next Step
When I revisited Dr. Pei and asked about using partially trained AI as a labeler with human review, he showed strong interest, but noted a major issue with a competing product: it’s hard to modify annotation content after AI labeling.
Our team is exploring this idea for significant cost savings. Research shows prompt tokens cost $0.00450 per 1,000, while manual annotation costs $0.01 per label.
AI-generated labels still need manual review, and the workflow is as follows:
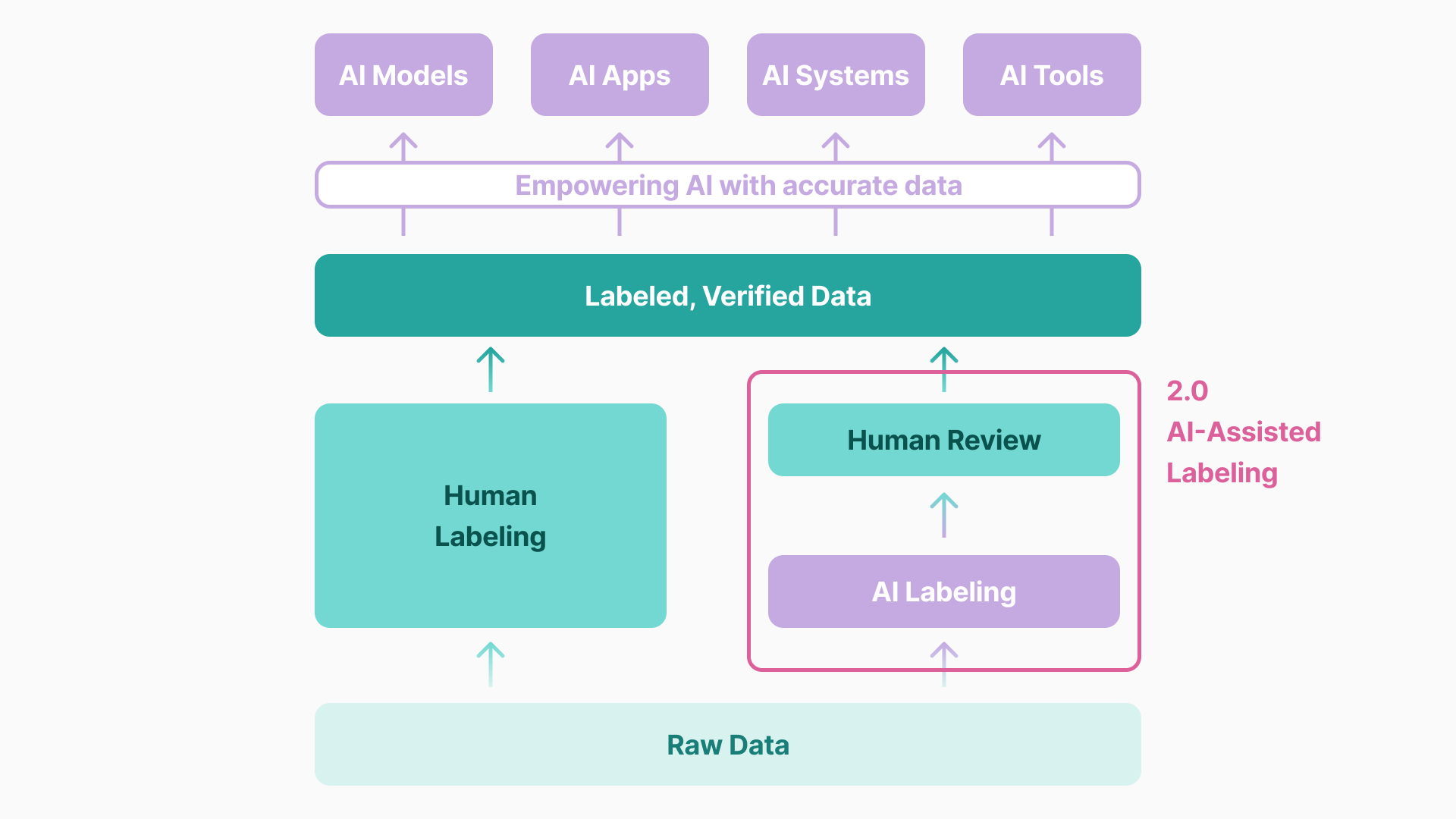
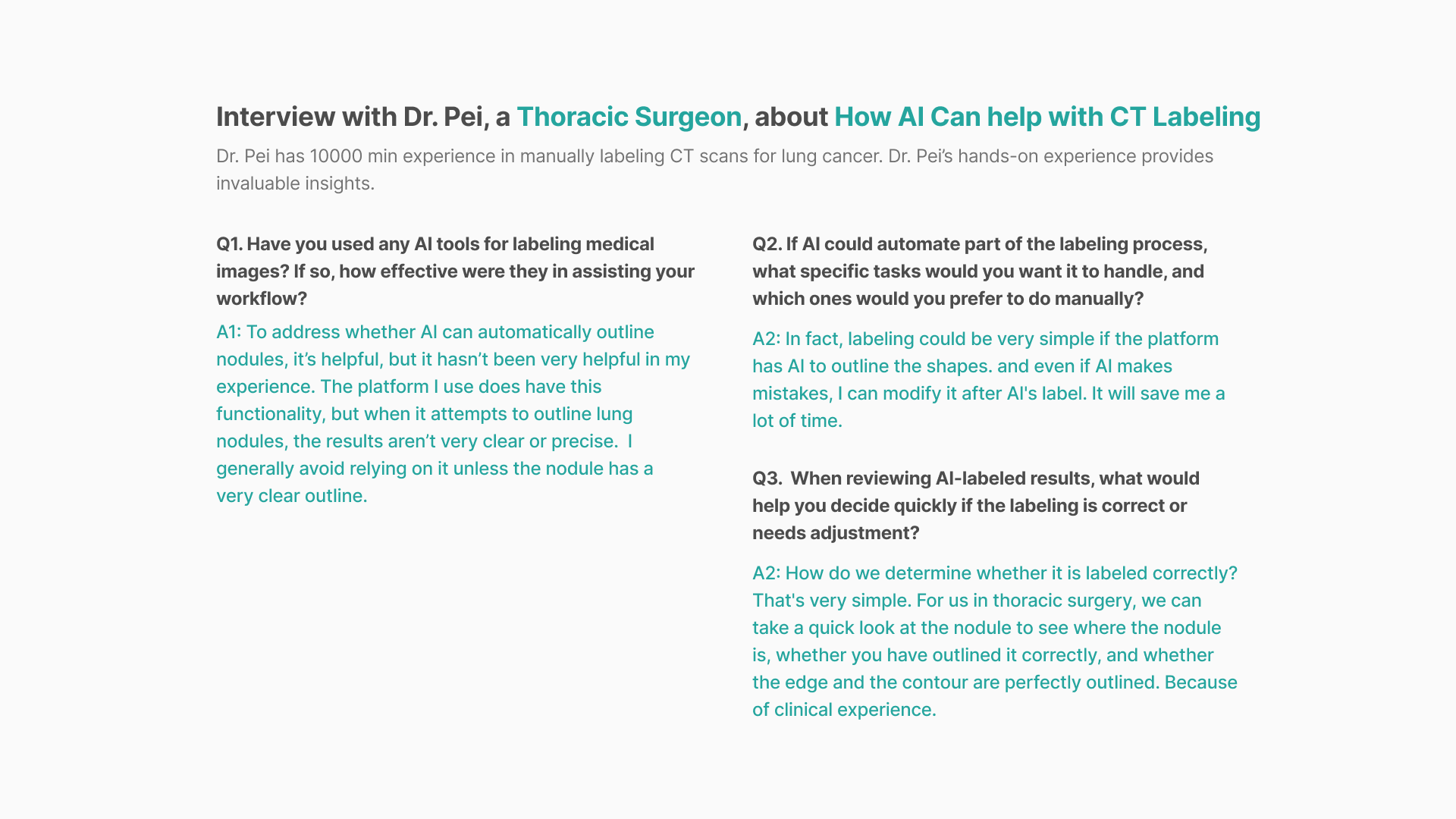
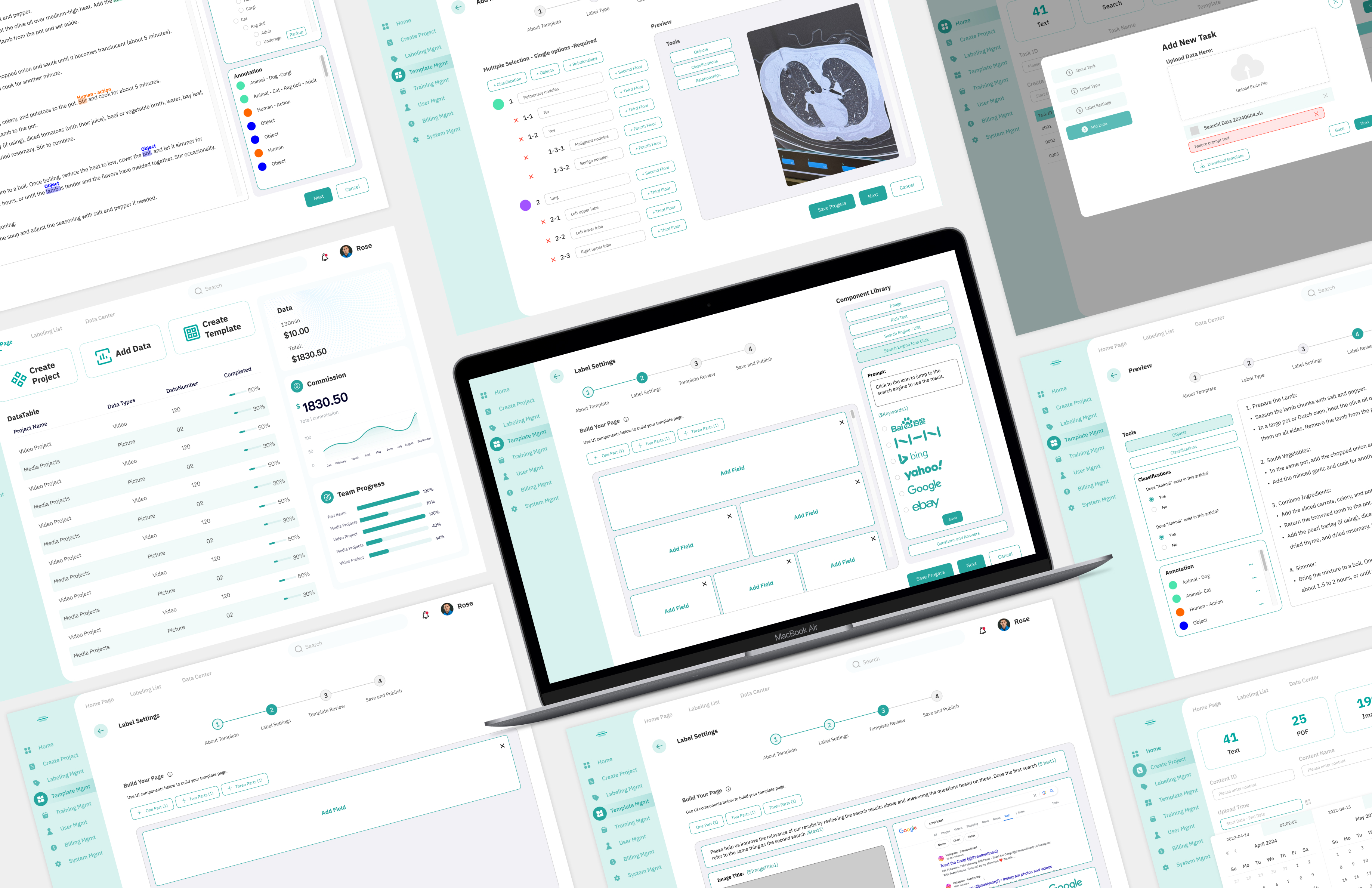